The A9 testfield is located near Garching. Overhead signs and sensor masts on the A9 freeway, the B 471 highway and at an intersection in Garching-Hochbrück are equipped with sensors that monitor traffic. In the four representations you can see the general overview (left), the virtual live twin of the traffic on the A9 (second box from the left), the live traffic on rural roads (second box from the right) and at the intersection with detected and classified road users (coloured boxes around the objects, right).
When displaying virtual traffic, there may be slight delays in building the image due to the large amount of data.
A9 Dataset: Scientific data from testfield A9 for download
As of now, data sets from test field A9 is available to the general public. The sets contain on the one hand labelled, time-synchronized and anonymized multi-modal sensor data covering area-scanning cameras, doppler radars, lidars and event-triggered cameras for a variety of traffic and weather-related scenarios, and on the other hand abstract digital twins of the traffic objects with position and trajectory-related information.
Providentia++: The testfield for autonomous and automated driving
The first phase of the Providentia project showed that the digital highway of the future is in principle possible. The challenge: Radar and lidar sensors installed in modern vehicles can survey the vehicle’s surroundings but have a limited range. In addition, they are not able to see behind passing vehicles. Providentia offers a “cross-vehicle system” that complements the vehicle’s own sensor technology.
During the first three years, it was shown that it is technically possible to create a digital twin of the traffic on the A9 highway in real time, using radar systems and cameras mounted on overhead highway signs. This virtual image can then be sent to any networked vehicle, thus increasing the range of the sensors in the vehicle. With apps, this fused data can also be used to make lane recommendations or warn of accidents.
FURTHER ARTICLES ABOUT THE PROJECT
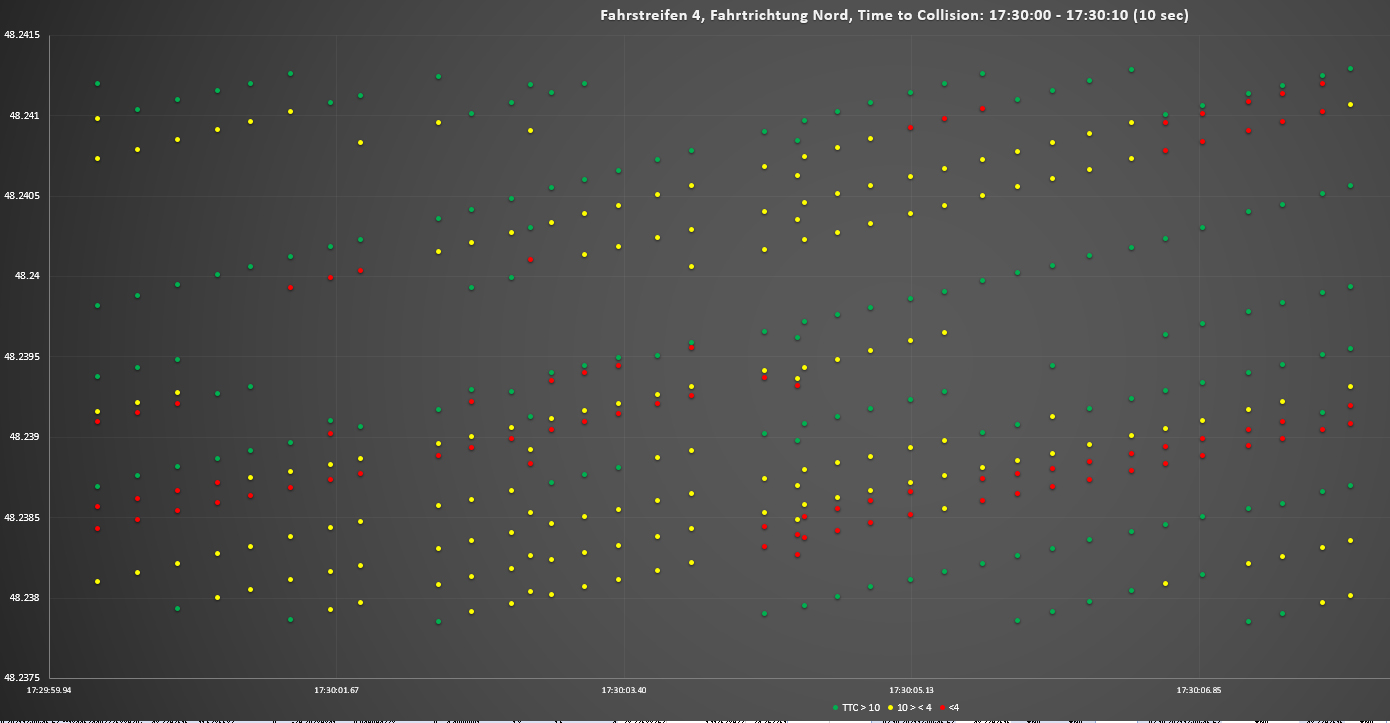
Data from the infrastructure: Managing traffic more precisely
Yunex uses the data from the A9 testfield to investigate Time to Collision in more detail. A field report.
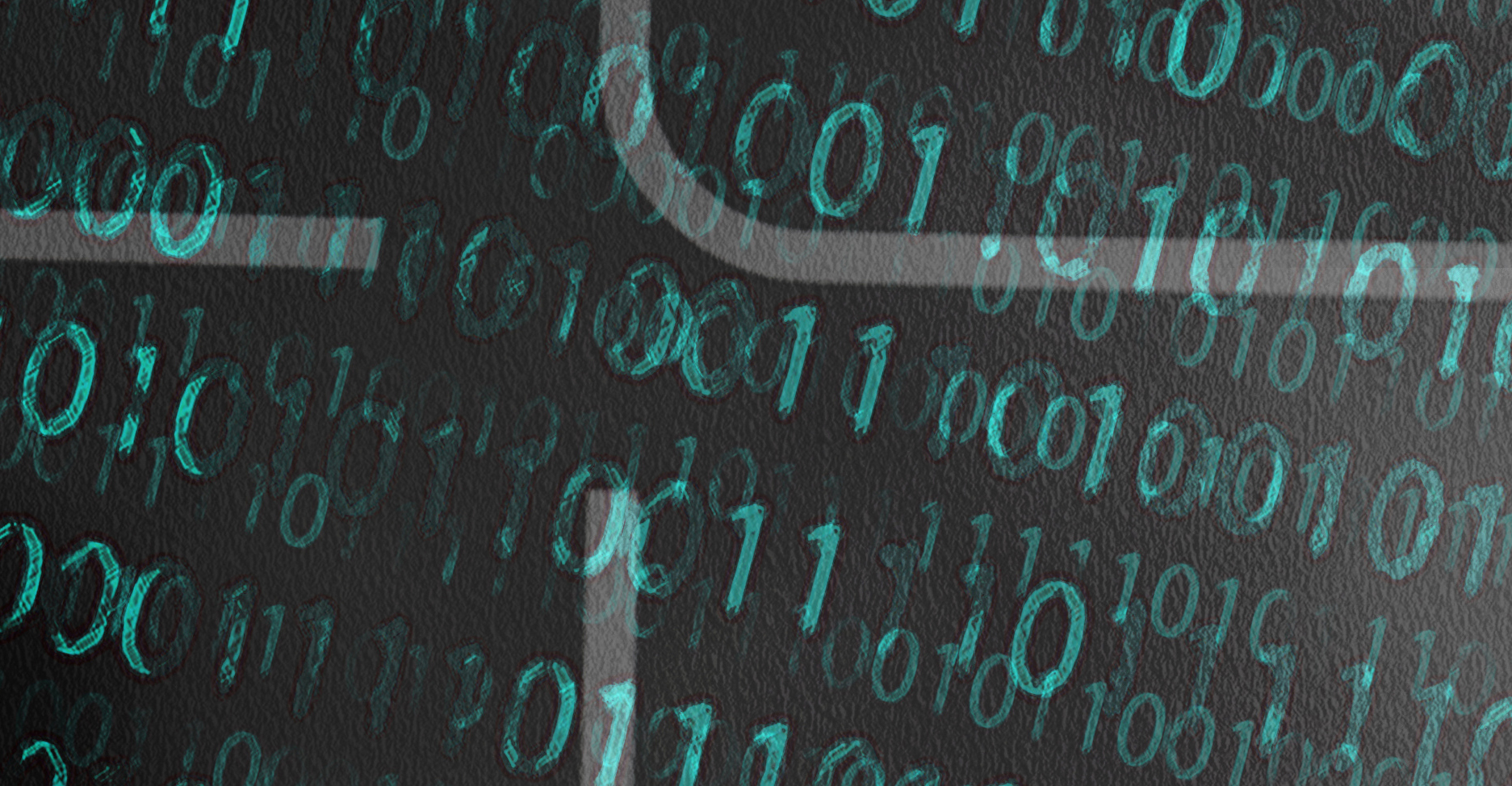
Traffic: Added value by big data
A huge amount of data is needed as to distinguish cars from motorcycles and bicyclists, to recognize maneuvers, to analyze accidents and to simulate traffic.
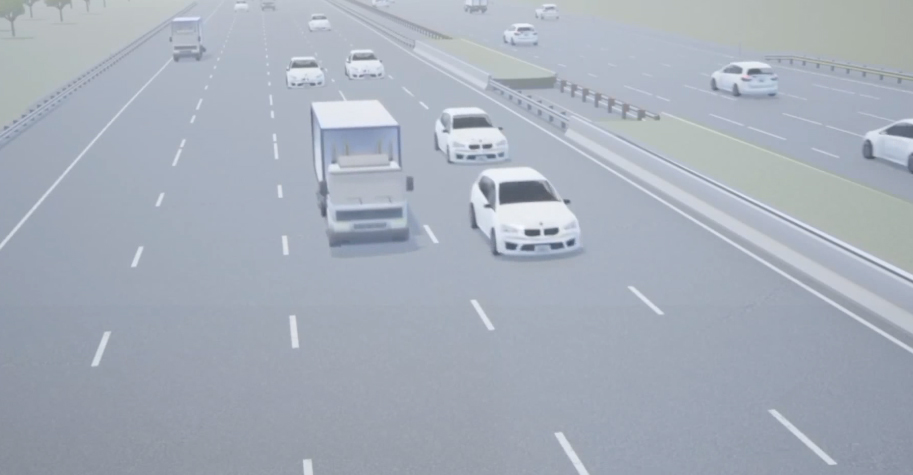
CARLA: What the most used driving simulator is capable of
The new CARLA simulation software can be used to run through scenarios and analyze traffic. Questions to the expert for 3-D object detection Walter Zimmer from the TU Munich.
Science: Focus on autonomous driving
Required for automated and autonomous driving: The bird's eye view for more safety
Providentia is an acronym formed from the German words for “Proactive Video-Based Use of Telecommunication Technologies in Innovative Traffic Scenarios.” Providentia is also the Roman goddess of providence and care. This is fitting, as the digital twin will make it possible for networked vehicles to predict which lane the vehicle should use in order to navigate through traffic as smoothly as possible and avoid traffic jams. It will also help prevent accidents, as the sensor technology will be able to anticipate hazards.
Providentia is an acronym formed from the German words for “Proactive Video-Based Use of Telecommunication Technologies in Innovative Traffic Scenarios.” Providentia is also the Roman goddess of providence and care. This is fitting, as the digital twin will make it possible for networked vehicles to predict which lane the vehicle should use in order to navigate through traffic as smoothly as possible and avoid traffic jams. It will also help prevent accidents, as the sensor technology will be able to anticipate hazards.
The first phase: Testing the digital twin on the highway
In the first phase of the Providentia project, high-resolution cameras and radar systems were mounted on two overhead signs on a test section of the A9 highway (no. 2 and 3 in the graph). The sensor data was transmitted wirelessly via 5G, artificial intelligence was used to identify vehicle types and classes, and the data was then “fused” – in other words, a digital twin was created. An autonomous vehicle was developed that can use the information from the digital twin to independently change lanes on the highway, for example, or to slow down to avoid traffic jams or accidents.
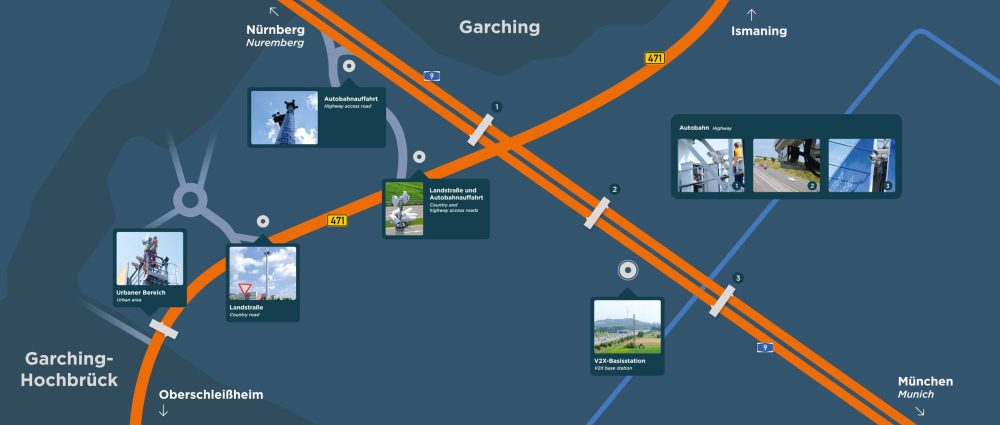
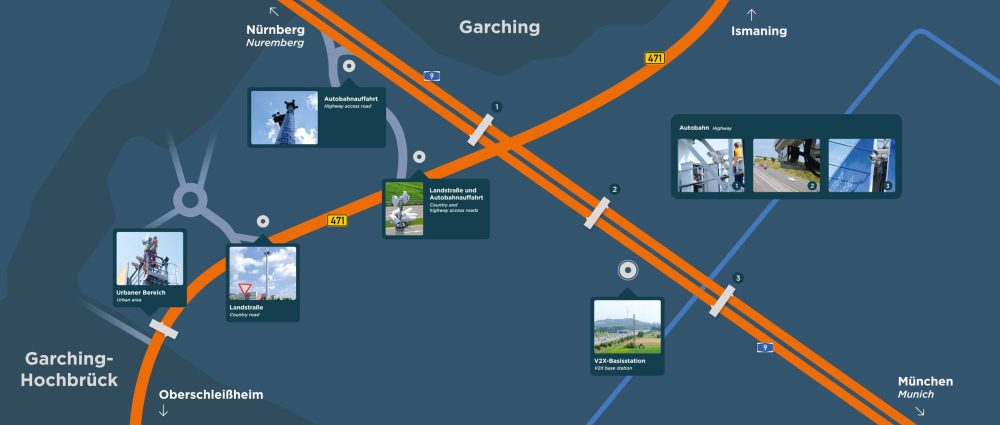
In the first phase of the Providentia project, high-resolution cameras and radar systems were mounted on two overhead signs on a test section of the A9 highway (no. 2 and 3 in the graph). The sensor data was transmitted wirelessly via 5G, artificial intelligence was used to identify vehicle types and classes, and the data was then “fused” – in other words, a digital twin was created. An autonomous vehicle was developed that can use the information from the digital twin to independently change lanes on the highway, for example, or to slow down to avoid traffic jams or accidents.
The second phase: From the highway to the residential area
By the end of 2019, the infrastructure on the A9 highway had been set up and the digital twin of the highway traffic had been developed. The next step: to fine-tune the technology, to expand the infrastructure into “urban space”, and to develop so-called value-added services. New transmitter and sensor towers will be erected on the edge of a feeder road into a residential area. Intersections, traffic circles, bus stops, train stations, and park-and-ride parking lots can be surveyed anonymously by the sensor technology.
Lidar systems, which generally cover a larger angle than radars, will be used in the project for the first time. In addition, value-added services will be created for drivers, highway operators, vehicle manufacturers, and academia. The follow-up project Providentia++ was launched in early 2020 and is expected to last two years. The current Providentia++ project is being funded with 9.16 million euros from the Federal Ministry of Transport and Digital Infrastructure (BMVI) and industry partners. The industry partners are the Technical University of Munich as consortium leader, Cognition Factory, Elektrobit Automotive, Valeo, fortiss, and Intel Deutschland. Additional associated partners are Huawei Technologies Deutschland, IBM Deutschland, brighter AI, and 3D Mapping Solutions.
4 Research areas for automated and autonomous driving
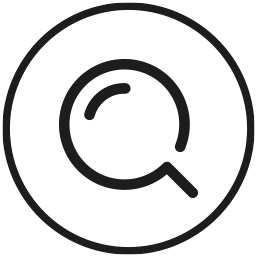
OBJECT RECOGNITION in multi-sensor scenarios
Data on the current traffic situation is constantly being recorded by eight area scan cameras and eight radars mounted on two overhead signs on the A9 highway. An area scan camera generates about 400 megabits per second, amounting to nearly 35 gigabytes per day. Since the systems should ultimately be as real-time capable as possible, a back-end computer reduces this volume of data to the essentials – information about the object and its class and position. In the end, only a few hundred bytes are generated per object. It is precisely this small volume that makes it possible to transfer the data in real time.
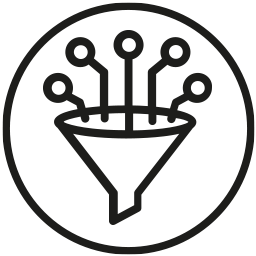
DATA FUSION and the digital twin
The goal is to uniquely identify objects and determine their position, with as little effort as possible. There are various challenges related to the fusion of sensor data: Cameras and radars record image data at different frequencies – cameras at 30 frames per second, and radars at 10 frames per second. They must be synchronized with each other. Moreover, sensor data is not always transmitted without interference. Vibrations on the overhead signs and poor weather conditions can impair data quality. The challenge is to evaluate and weight the individual data sources, thus laying the foundation for local digital twins, which are created at the respective measuring stations, and global twins, which take all available sensor data into account.
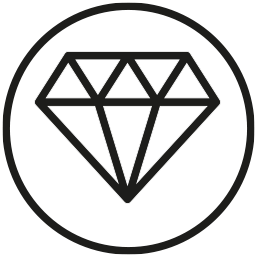
ERROR TOLERANCE, reliability, and scalability
If individual sensors provide poor data or even fail, the overall system must still be able to provide reliable information. The goal is for the overall system to be able to decide autonomously which systems are used and which are left out of the calculations. This makes the digital twin error-tolerant and able to provide usable information at all times for vehicles that use value-added services from the digital twin. It is also interesting that in-vehicle assistance systems are able to check their own sensor data for plausibility via the external infrastructure. This makes the digital twin even more reliable. Warning of risk of collision, improving traffic flow, controlling traffic, and enabling real-time traffic analysis: These are services that are of interest to very different stakeholders, including vehicle owners, highway operators, and car manufacturers.
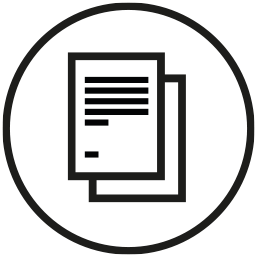
COMMUNICATION PROTOCOL
Data exchange between system units and vehicles in real time necessitates customized message protocols that adapt the available network capacity to the specific situation and that prioritize tasks. Every vehicle that is near the overhead highway signs receives customized information – in other words, its individual digital twin. If two vehicles from the same “region of interest” request a digital twin at the same time, they receive it via broadcast, otherwise via unicast. The assignment has to be accurate.
Data fusion, prediction, V2X: Our scientific publications
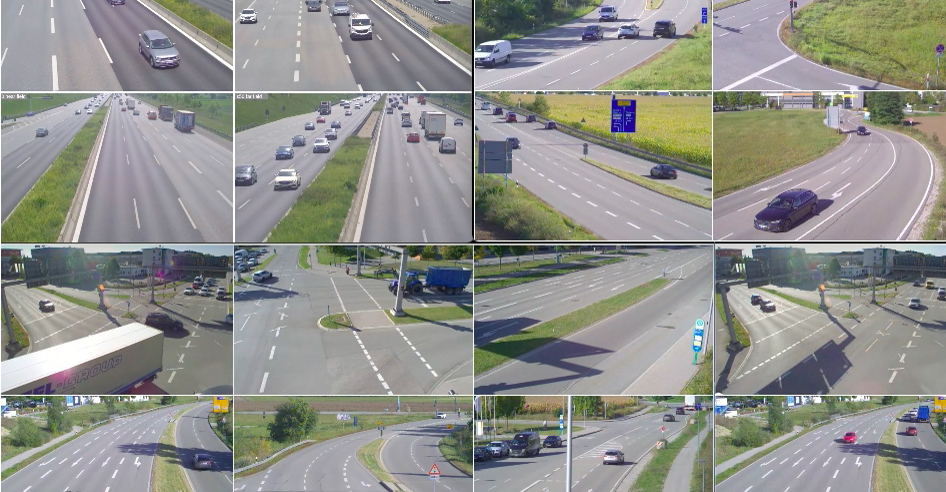
Comparison of test fields with external infrastructure for automated and autonomous driving
Intelligent Transport Systems (ITS) with external infrastructure: 350 global publications and 40 test sites for automated and autonomous driving compared.

Predicting movements by autonomous agents
To predict movements, many trajectory options must be taken into consideration and prioritized. The FloMo model works with probabilities and has been trained on the basis of three datasets. Its performance is improved by a proposed method of stabilizing training flows.
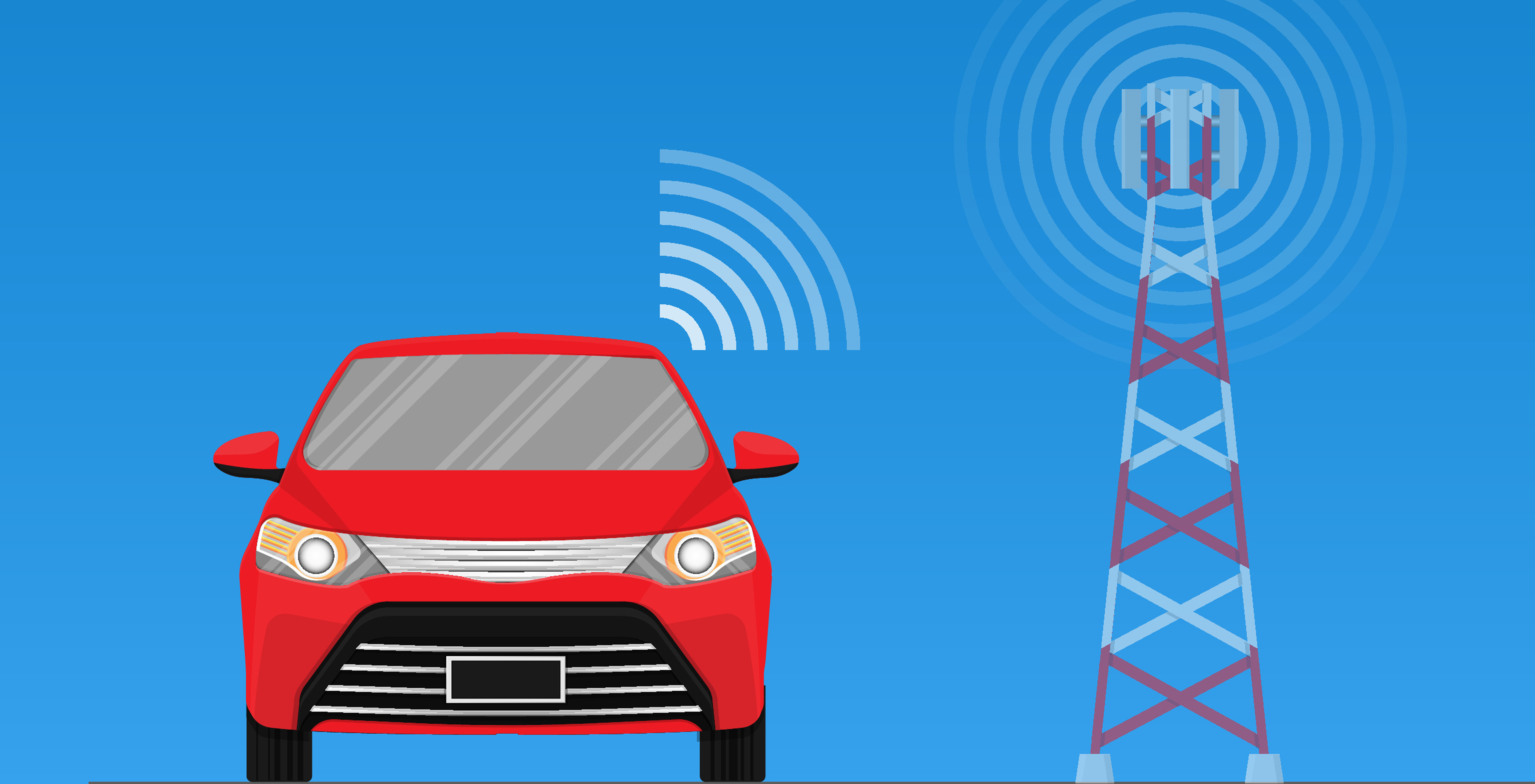
C-V2X: Architecture for deployment in moving network convoys
Reliable V2X communication is crucial for the development of cooperative intelligent transportation systems (ITS) – and for improving traffic safety and efficiency. A research topic of Providentia++: The approach of moving network convoys.
"A development toward autonomous vehicles can take place very quickly."
The better sensors from vehicles and infrastructure are networked, the more seamlessly the traffic area can be recorded. This ultimately benefits not only motorists, but all other road users as well. Mobility expert Prof. Dr. Alois Knoll and his vision of the mobility of the future.
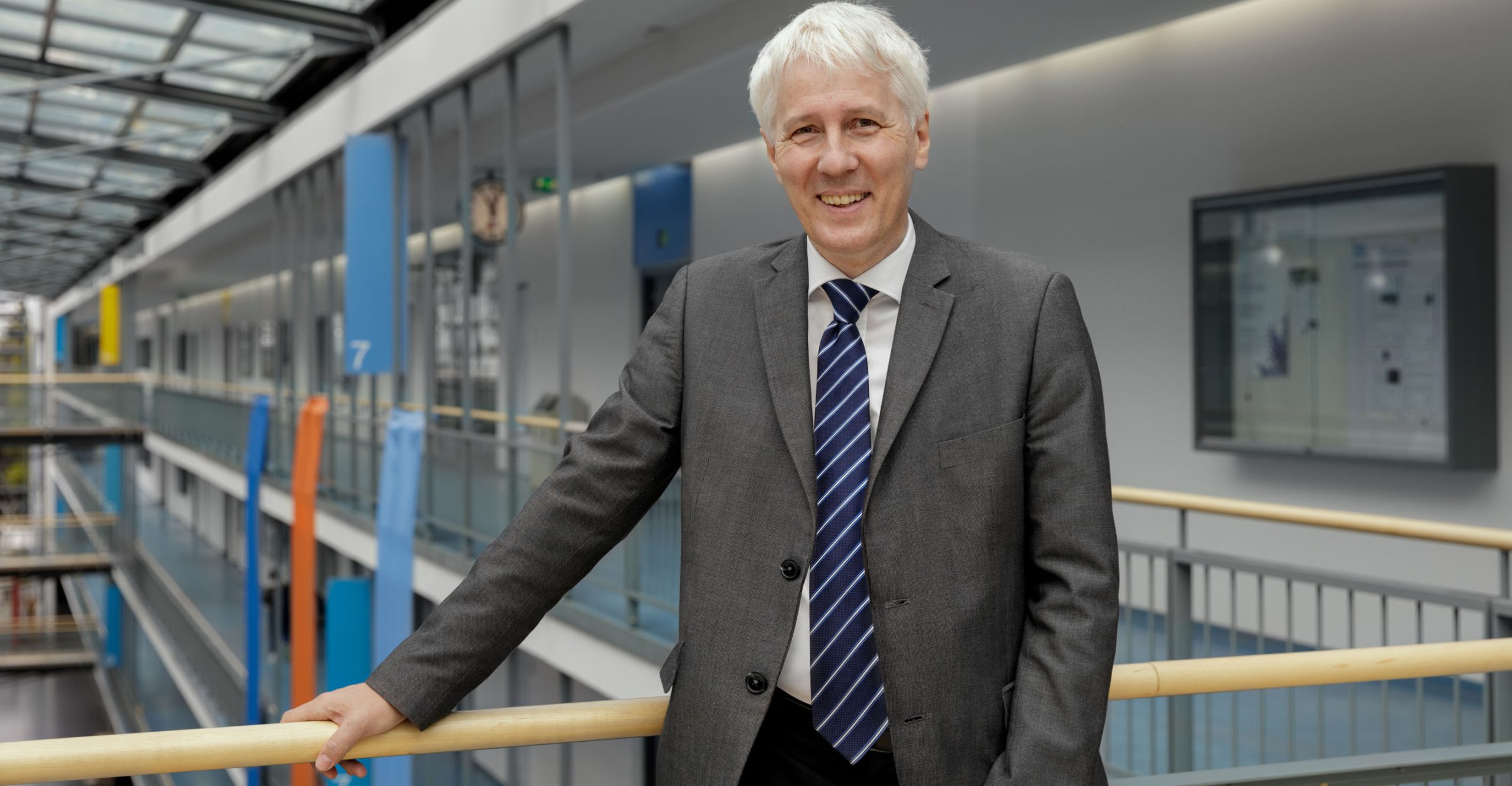
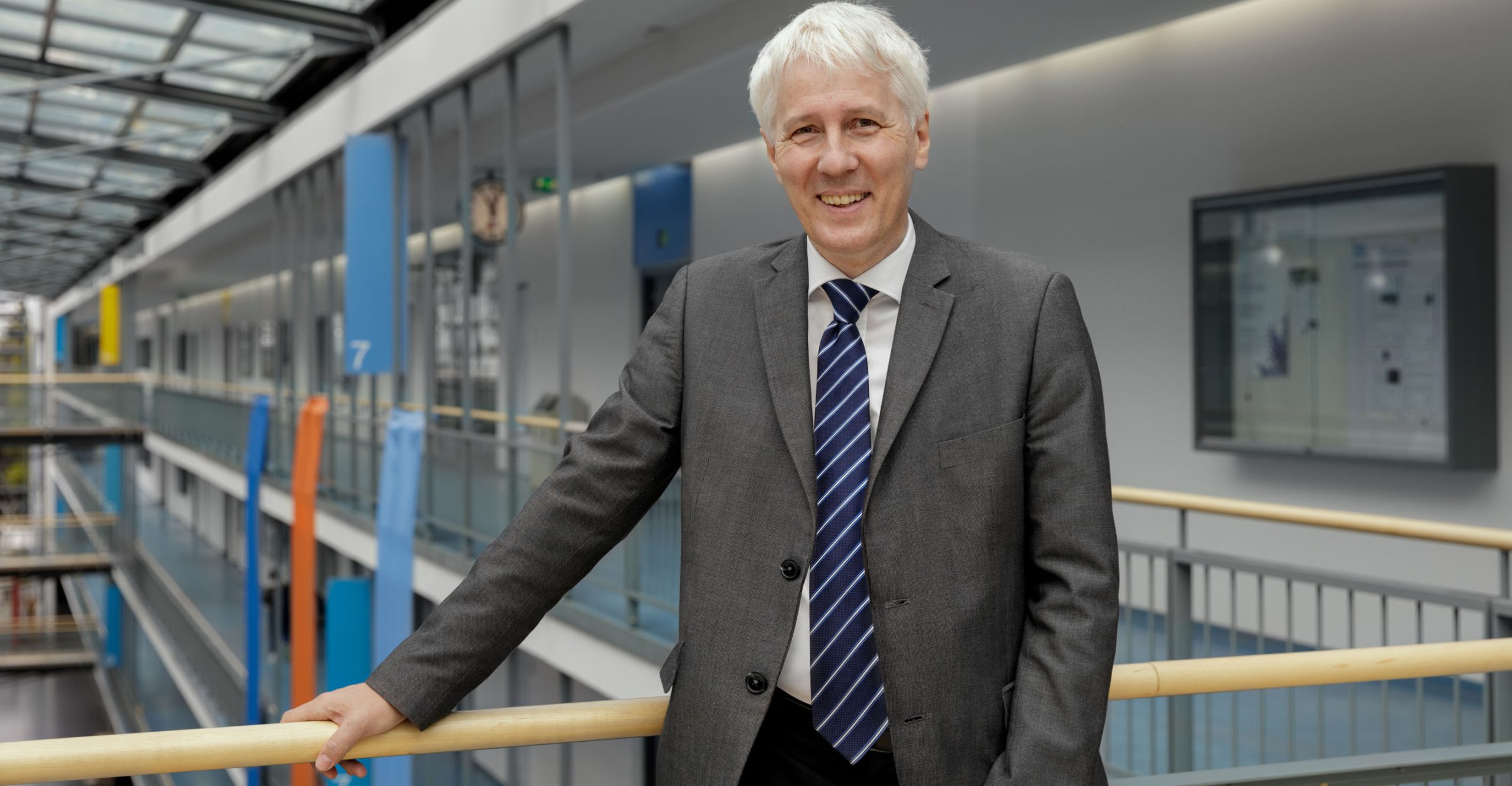
The better sensors from vehicles and infrastructure are networked, the more seamlessly the traffic area can be recorded. This ultimately benefits not only motorists, but all other road users as well. Mobility expert Prof. Dr. Alois Knoll and his vision of the mobility of the future.
PROVIDENTIA TEAM
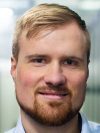
Bernhard Blieninger, Scientist, fortiss
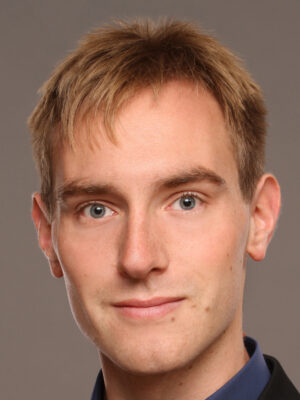
Christian Creß, Scientist, TU Munich
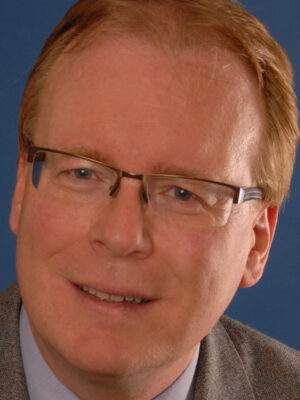
Markus Dillinger, Director 5G R&D, Huawei

Marian Gläser, CEO, brighter AI

Ralf Gräfe, Program Manager, Intel Labs Europe

Dr.-Ing. Gunnar Gräfe, CEO, 3D Mapping Solutions
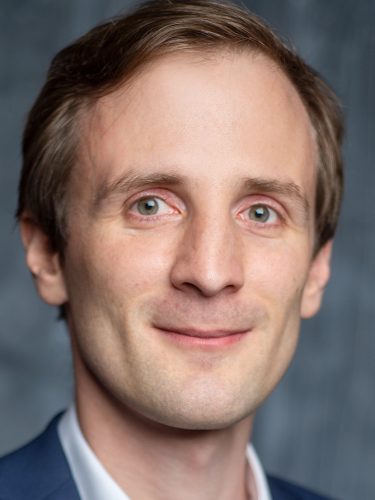
Gereon Hinz, Visiting Scientist, STTech
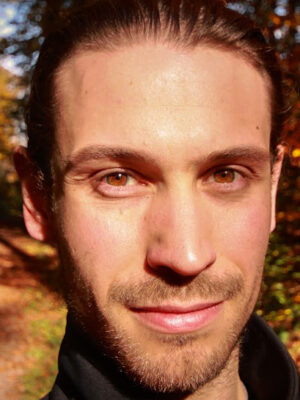
Dr. Jens Honer, Senior Expert, Valeo
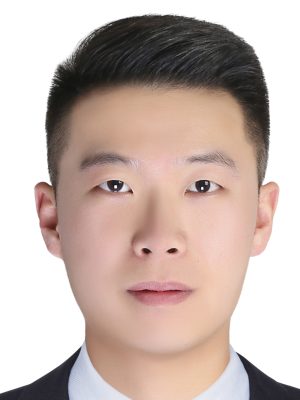
Xinyi Li, Scientist, TU Munich
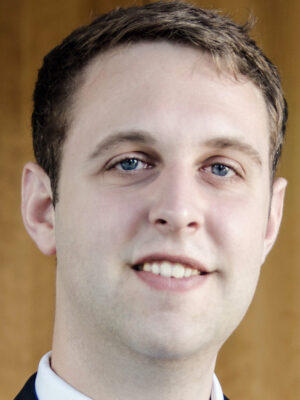
Juri Kuhn, Scientist, fortiss
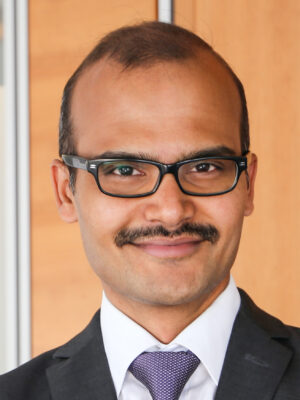
Venkatnarayanan Lakshminarasimhan, Scientist, TU Munich
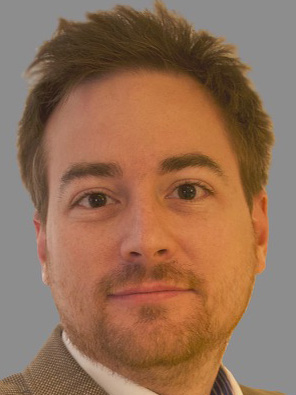
Dr. Claus Lenz, CEO, Cognition Factory
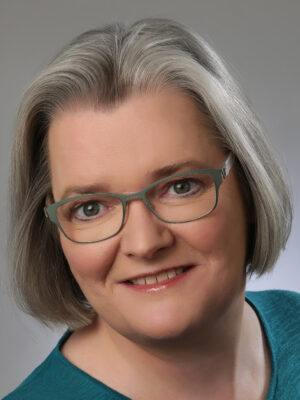
Marie-Luise Neitz, Manager Third-Party Funded Projects, TU Munich
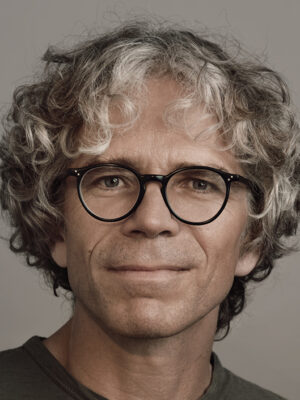
Andreas Schmitz, Public and Media Relations, TU Munich
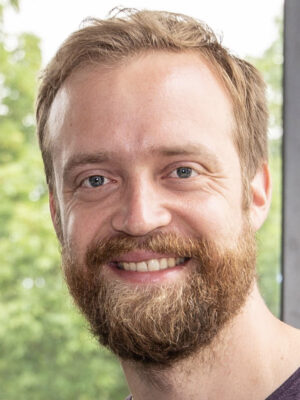
Christoph Schöller, Scientist, fortiss
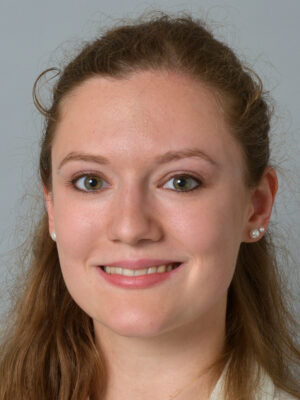
Leah Strand, Scientist, TU Munich
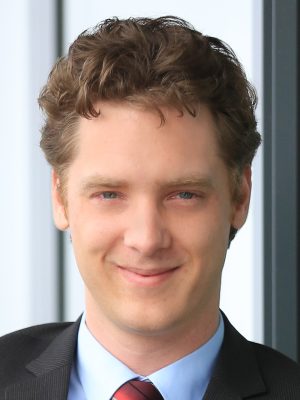
Simon Tiedemann, Senior Product Manager, Elektrobit
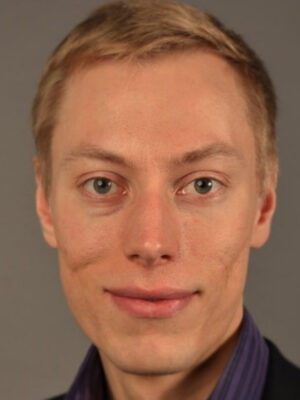
Walter Zimmer, Scientist, TU Munich
PARTNERS
ASSOCIATED PARTNERS
FUNDING
PROJECT SPONSOR
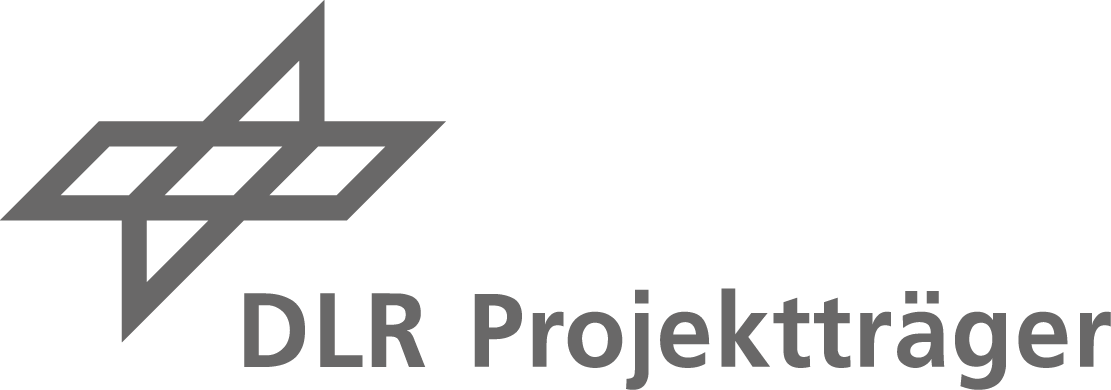